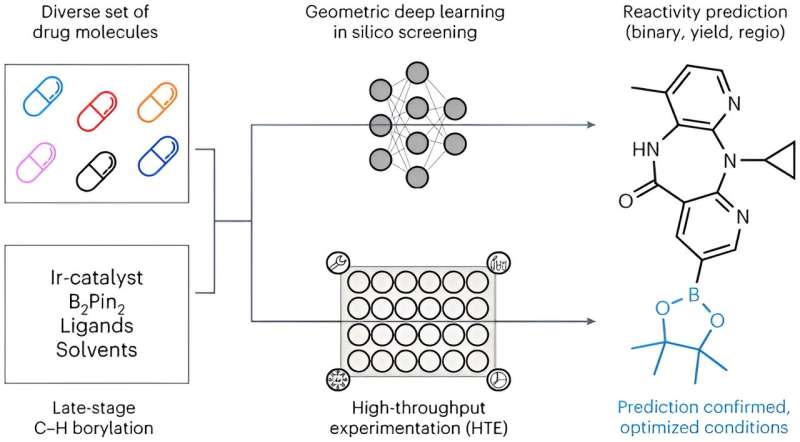
New active pharmaceutical ingredients lay the foundations for innovative and better medical treatments. However, identifying them and, above all, producing them through chemical synthesis in the laboratory is no mean feat. To home in on the optimum production process, chemists normally use a trial-and-error approach: they derive possible methods for laboratory synthesis from known chemical reactions and then test each one with experiments, a time-consuming approach that is littered with dead ends.
Now, scientists at ETH Zurich, together with researchers from Roche Pharma Research and Early Development, have come up with an approach based on artificial intelligence that helps to determine the best synthesis method, including its probability of success. Their paper is published in the journal Nature Chemistry.
"Our method can greatly reduce the number of lab experiments required," explains Kenneth Atz, who developed the AI model as a doctoral student together with Professor Gisbert Schneider at the Institute of Pharmaceutical Sciences at ETH Zurich.
Active pharmaceutical ingredients usually consist of a scaffold onto which are bound what are known as functional groups. These are what give the substance its highly specific biological function. The scaffold's job is to bring the functional groups into a defined geometric alignment so that they can act in a targeted manner. Imagine a crane construction kit, in which a framework of connecting elements is bolted together in such a way that functional assemblies like rollers, cable winches, wheels and the driver's cab are arranged correctly in relation to each other.
Introducing chemical functions
One way to produce drugs with a new or improved medicinal effect involves placing functional groups at new sites on the scaffolds. This might sound simple, and it certainly wouldn't pose a problem on a model crane, but it is particularly difficult in chemistry. This is because the scaffolds, being primarily composed of carbon and hydrogen atoms, are themselves practically nonreactive, making it difficult to bond them with functional atoms such as oxygen, nitrogen or chlorine. For this to succeed, the scaffolds must first be chemically activated via detour reactions.
One activation method that opens up a great many possibilities for different functional groups, at least on paper, is borylation. In this process, a chemical group containing the element boron is bonded to a carbon atom in the scaffold. The boron group can then simply be replaced by a whole range of medically effective groups.
Data from trustworthy sources and an automated lab
"Although borylation has great potential, the reaction is difficult to control in the lab. That's why our comprehensive search of the worldwide literature only turned up just over 1,700 scientific papers on the subject," Atz says, describing the starting point for his work.
The idea was to take the reactions described in the scientific literature and use them to train an AI model, which the research team could then use to consider new molecules and identify as many sites as possible on them where borylation would be feasible. However, the researchers ultimately fed their model only a fraction of the literature they found. To ensure that the model wasn't misled by false results from careless research, the team limited itself to 38 particularly trustworthy papers. These described a total of 1,380 borylation reactions.
To expand the training dataset, the team supplemented the literature results with evaluations of 1,000 reactions carried out in the automated laboratory operated by Roche's medicinal chemistry research department. This allows many chemical reactions to be carried out at the milligram scale and analyzed simultaneously.
"Combining laboratory automation with AI has enormous potential to greatly increase efficiency in chemical synthesis and improve sustainability at the same time," says David Nippa, a doctoral student from Roche who accomplished the project together with Atz.
High predictive power, especially with 3D data
The predictive capabilities of the model generated from this data pool were verified using six known drug molecules. In 5 out of 6 cases, experimental testing in the laboratory confirmed the predicted additional sites. The model was just as reliable when it came to identifying sites on the scaffold where activation isn't possible. What's more, it determined the optimum conditions for the activation reactions.
Interestingly, the predictions got even better when 3D information on the starting materials was included rather than just their two-dimensional chemical formulas. "It seems the model develops a kind of three-dimensional chemical understanding," Atz says.
The success rate of the predictions also impressed the researchers at Roche Pharma Research and Early Development. In the meantime, they have successfully used the method to identify sites in existing drugs where additional active groups can be introduced. This helps them to develop new and more effective variants of known active pharmaceutical ingredients more quickly.
Sights set on other activations and functionalizations
Atz and Schneider see numerous other possible applications for AI models that are based on a combination of data from trustworthy literature and from experiments conducted in an automated laboratory. For instance, this approach ought to make it possible to create effective models for activation reactions other than borylation. The team is also hoping to identify a wider range of reactions for further functionalizing the borylated sites.
Atz is now involved in this further development work as an AI scientist in medicinal chemistry research at Roche. "It is very exciting to work at the interface of academic AI research and laboratory automation. And it is a pleasure to be able to drive this forward with the best content and methods," says Atz.
Schneider adds, "This innovative project is another outstanding example of collaboration between academia and industry and demonstrates the enormous potential of public-private partnerships for Switzerland."
More information: David F. Nippa et al, Enabling late-stage drug diversification by high-throughput experimentation with geometric deep learning, Nature Chemistry (2023). DOI: 10.1038/s41557-023-01360-5
Provided by ETH Zurich
Citation: New AI model identifies new pharmaceutical ingredients and improves existing ones (2023, November 24) retrieved 24 November 2023 from https://ift.tt/7enANRa
This document is subject to copyright. Apart from any fair dealing for the purpose of private study or research, no part may be reproduced without the written permission. The content is provided for information purposes only.
"ingredients" - Google News
November 24, 2023 at 09:18PM
https://ift.tt/7aviRFL
New AI model identifies new pharmaceutical ingredients and improves existing ones - Phys.org
"ingredients" - Google News
https://ift.tt/rAuFdmO
Shoes Man Tutorial
Pos News Update
Meme Update
Korean Entertainment News
Japan News Update
Bagikan Berita Ini
0 Response to "New AI model identifies new pharmaceutical ingredients and improves existing ones - Phys.org"
Post a Comment